摘要:当前国际进化生物学与生态学研究的主流分子标记数可达数以百计甚至上千,但以昆虫为代表的无脊椎动物大部分类群仍缺乏类群特异性参考分子标记。本文提出了一套开发通用系统发育分子标记的分析流程,该流程基于二代全基因组测序 (Whole-genome sequencing, WGS),使用一系列高效的生物信息学工具快速组装和注释基因组,设计目标类群的通用单拷贝核基因 (Universal Single-Copy Orthologs, USCOs) 和超保守元件 (Ultraconserved Elements, UCEs) 标记,并分别产生兼容通用单拷贝核基因 (Benchmarking Universal Single-Copy Orthologs, BUSCO) 参考数据集的格式以及开发相应的杂交富集探针,以方便下游分子标记的提取和后续分析。本流程得到基因组实测数据的有力支持,为相应研究提供了快速有效的方法与途径。
关键词: 全基因组测序, 高通量测序, 通用单拷贝核基因, 超保守元件, 系统发育基因组学
研究背景
高通量测序产生的基因组规模的数据集有利于解决进化生物学和生态学中各种具有挑战性的问题。基因组标记 (Genomic partitioning) 策略是目前主要的数据收集方法,用于富集特定基因组区域的序列,例如,转录组和杂交富集。高通量测序成本快速降低进一步推动了产生有/无参考基因组的分子标记开发。然而,对于部分动物类群来说,全面的基因组资源十分有限。在无脊椎动物中,几乎没有类群具有大量参考基因组和注释信息,基因标记或用于富集靶向基因标记的探针也很少,这严重阻碍了基因组规模数据集的产生和下游分析。
单拷贝核基因和超保守元件是系统学界广泛应用的系统发育基因组学标记,并已被应用于多种生物的不同分类阶元。然而,在大多数中、低阶元的类群中没有参考单拷贝同源序列,只有更高阶元的基准通用单拷贝同源序列集,详见BUSCO官网 (https://busco.ezlab.org/)。此外,可用的超保守元件探针只存在于几个较大的昆虫目中,如鞘翅目、双翅目、半翅目和膜翅目 (https://www.ultraconserved.org/)。针对这一现状,本研究开发了一套新的流程来加速开发相应的分子标记。
本文提供了详细的利用基因组数据设计通用单拷贝核基因与超保守元件分子标记的分析流程,该方案的有效性已在弹尾纲的分子系统学研究中得以验证,详细内容请参阅Sun et al., 2020。
仪器设备
- 普通电脑 (8核16线程,内存:32 G;可在Windows系统预先安装好虚拟机VMware或VirtualBox)。
软件
- 基因组组装
BBTools v38.90 (https://sourceforge.net/projects/bbmap/)
Minia v3.2.4 (https://github.com/GATB/minia)
Redundans v0.14c (https://github.com/lpryszcz/redundans)
Minimap2 v2.17 (https://github.com/lh3/minimap2)
BESST v2.2.8 (https://github.com/ksahlin/BESST)
GapCloser v1.12 (http://soap.genomics.org.cn/)
BUSCO v3.0.2 (https://busco.ezlab.org/)
SPAdes v3.14.1 (http://bioinf.spbau.ru/spades)
- 基因组注释
WindowMasker (https://github.com/goeckslab/WindowMasker)
BRAKER2 (https://github.com/Gaius-Augustus/BRAKER)
DIAMOND (https://github.com/bbuchfink/diamond)
CD-HIT (http://weizhongli-lab.org/cd-hit/)
BUSCO v3.0.2 (https://busco.ezlab.org/)
- BUSCO数据集设计
OrthoFinder v2.2.7 (https://github.com/davidemms/OrthoFinder)
MAFFT v7.394 (https://mafft.cbrc.jp/alignment/software/)
HMMER v3.2.1 (http://hmmer.org/download.html)
- UCE设计
faToTwoBit (http://hgdownload.soe.ucsc.edu/admin/exe/)
ART-20160605 (https://www.niehs.nih.gov/research/resources/software/biostatistics/art/index.cfm)
Stampy v1.0.32 (http://www.well.ox.ac.uk/project-stampy)
Samtools v1.7 (http://www.htslib.org/)
BEDTools v2.29.0 (https://github.com/arq5x/bedtools2)
PHYLUCE v1.6.6 (http://phyluce.readthedocs.io/en/latest/index.html)
实验步骤
该部分主要指利用高通量二代测序原始数据或已发表的基因组,设计目标类群的特异性参考分子标记,具体流程见图1。
一、数据获取
分析数据来源于Illumina测序的全基因组原始数据或从GenBank等相关数据库获得已公开发表的基因组数据。该方案摘自本课题组2020年发表在Molecular Ecology Resources上的文章:"Streamlining universal single-copy orthologue and ultraconserved element design: A case study in Collembola",并以文章中的数据作为测试数据 (Sun et al., 2020)。
二、基因组组装
低深度二代测序的基因组组装建议使用Zhang et al. (2019) 的PLWS快速流程 (https://github.com/xtmtd/PLWS),该流程可以从低深度测序的基因组数据中快速从头组装基因组,单个物种基因组 (基因组大小0.1-2 Gbp) 在台式电脑上仅用时2-24 h完成组装。组装流程包括原始序列质控、降低丰度 (BBTools; Bushnell, 2014)、组装 (Minia3; Chikhi and Rizk, 2013)、去杂合 (Redundans; Pryszcz and Gabaldón, 2016)、序列延长 (BESST; Sahlin et al., 2014)、补洞 (GapCloser; Luo et al., 2012),详见https://github.com/xtmtd/PLWS/blob/master/script1_Genome_assembly.sh。如果计算资源充分,也可以使用Spades、Abyss等二代组装工具,以SPAdes v3.14 (Bankevich et al., 2012) 为例:
使用的代码:spades.py -1 FORWARD.fastq.gz -2 REVERSE.fastq.gz -o $OUT_FOLDER -t $THREADS
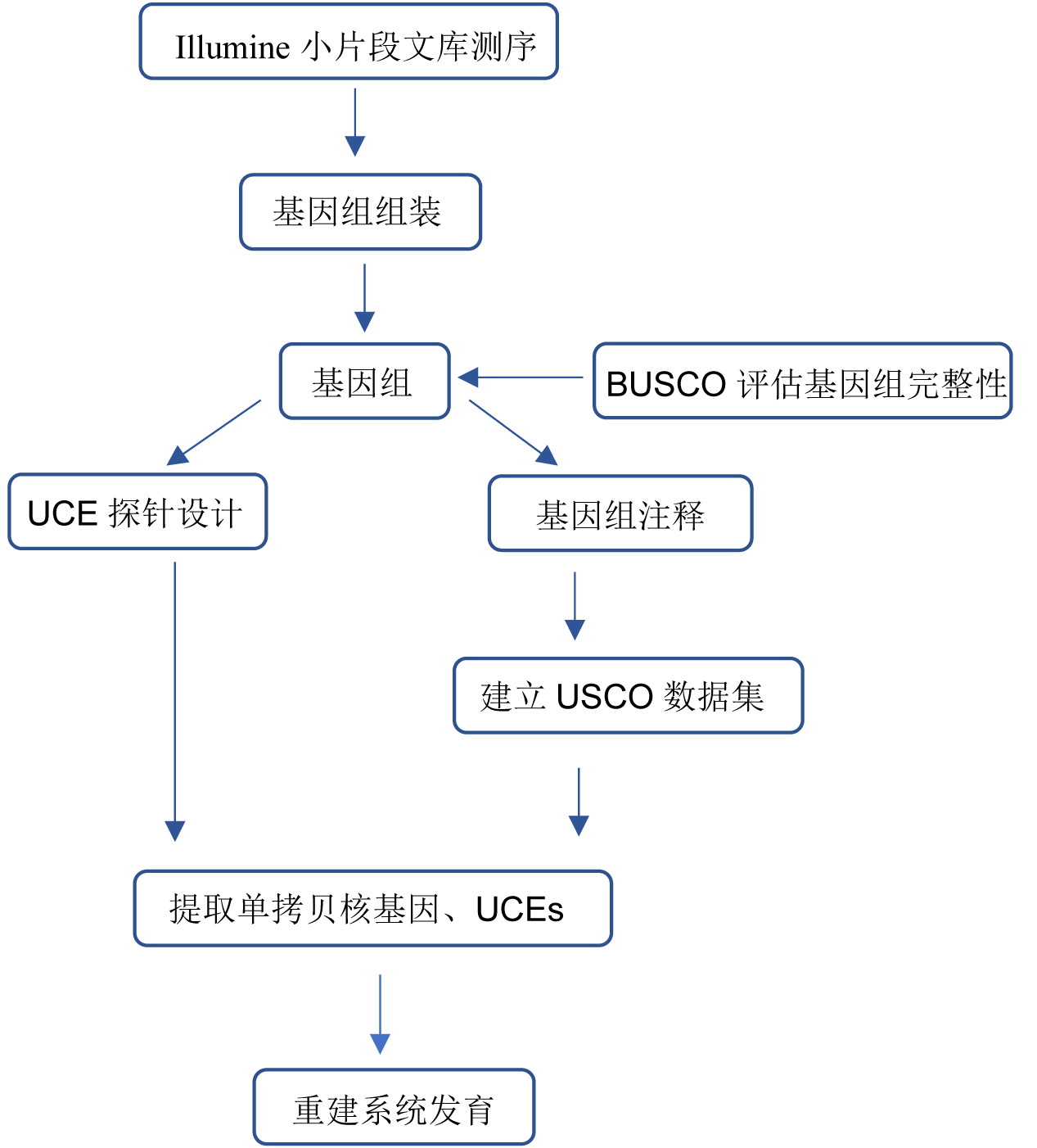
图1. 基因组数据设计通用单拷贝核基因与超保守元件分子标记流程图
三、基因组注释
- 重复序列屏蔽。基因组中的重复序列会导致BLAST的结果出现假阳性,增加基因结构预测的计算压力,甚至影响注释正确性。建议使用WindowMasker (Morgulis et al., 2006) 屏蔽基因组重复序列,该软件不需要构建重复序列文库,可以根据基因组序列本身快速识别并标记高重复序列和低复杂度序列。
使用的代码:windowmasker -mk_counts -in genome.$SPECIES.fa -out masking_library.ustat -mem 60000
windowmasker -ustat masking_library.ustat -dust T -in genome.$SPECIES.fa -out genome.masked.fa -outfmt fasta
注意:windowmasker的详细参数和运行介绍可参阅https://github.com/goeckslab/WindowMasker - 基因注释。基因预测通常是需要整合从头预测、转录组或蛋白同源证据,如MAKER或evm流程。然而,非模式生物通常缺乏转录组或蛋白质数据。建议使用BRAKER2 (Bruna et al., 2015) 进行基因预测,它可以在没有外部证据,即转录组、蛋白同源证据的情况下完全自动地预测蛋白质编码基因。由于从Illumina数据组装的基因组存在片段化现象,本文中建议注释时设置最小序列长度为20,000 bp,蛋白数据库odb.fa来源于OrthoDB。最终注释的蛋白序列保存 augustus.hints.aa。
使用的代码:braker.pl --genome=genome.masked.fa --prot_seq=/path/proteins_ref/odb.fa --epmode --softmasking --species=$SPECIES --cores $CORES --min_contig=20000
注意:对于没有或不需要近缘序列作为输入的,可以删除参数--prot_seq,BRAKER2的详细参数和运行介绍可参阅https://github.com/Gaius-Augustus/BRAKER。 - 蛋白比对。将注释出的候选蛋白序列,与直同源蛋白数据库进行比对,将未成功匹配的同源蛋白序列视为非候选蛋白序列。此步建议使用DIAMOND (Buchfink, Xie and Huson, 2015),它对蛋白质和翻译后的核苷酸的比对速度是BLAST的100-10,000倍。首先,创建diamond格式的数据库文件;其次,在blastp模式下进行搜索;最后,根据比对上的基因id提取最终的蛋白序列。
使用的代码和参数:diamond makedb --in odb.fa -d odb
diamond blastp -d /path/proteins_ref/odb -q augustus.hints.aa -o blastp.out -f 6 --sensitive -p 32 -e 1e-5 --max-target-seqs 1
cat blastp.out | awk '{print $1}' | sort | uniq > gene.braker.list
cat augustus.hints.aa | seqkit grep -f gene.braker.list > proteins.$SPECIES.fa
注意:DIAMOND的详细参数和运行介绍可参阅https://github.com/bbuchfink/diamond。 - 去除冗余蛋白序列。建议使用CD-HIT (Fu et al., 2012),可以根据蛋白质序列或核酸序列的相似性对序列进行聚类以去除冗余的序列,生成非冗余的数据集用于后续的实验分析。该软件速度快,而且适用于大数据。
使用的代码和参数:cd-hit -i proteins.$SPECIES.fa -o proteins.$SPECIES.cdhit.fa -c 0.97 -n 5 -M 28000 -d 0 -T $THREADS
注意:CD-HIT的详细参数和运行介绍可参阅http://weizhongli-lab.org/cd-hit/。 - 评估注释的蛋白序列完整性。
使用的代码:run_BUSCO.py -i proteins.$SPECIES.cdhit.fa -m prot -c $THREADS -o protein -l /path/busco-3.0.2/lineage/arthropoda_odb9/
注意:BUSCO (Simão et al., 2015) 的详细参数和运行介绍可参阅https://busco.ezlab.org/。
四、BUSCO设计
- 建议选择组装质量较好 (基因组BUSCO完整性评估> 80%) 的基因组用于分子标记开发。并将所有注释的蛋白质序列复制到同一文件夹中,比如proteins,使用OrthoFinder (Emms and Kelly, 2019) 寻找直系同源基因家族。
使用的代码和参数:orthofinder -f proteins -t $THREADS -a 1 -S diamond -os -M msa
注意:OrthoFinder软件的详细参数和运行介绍可参阅https://github.com/davidemms/OrthoFinder。
- 根据OrthoFinder的结果提取所有物种共有的近通用单拷贝基因信息,对于单个基因,所有物种只允许1次基因缺失或基因复制,相应的直同源基因被提取作为USCO候选基因,基因编号保存在candidates.list文档中,并提取candidates.list中的直同源基因,最后将提取的每个基因的蛋白序列保存在文件夹candidates_seqs中,用于后续分析。
- 使用MAFFT (Katoh and Standley, 2013) 对提取的每个基因进行单独比对。
使用的代码:linsi --thread $THREADS --anysymbol /path/candidates_seqs/$gene.fa > $gene.mafft.fa
注意:MAFFT软件的详细参数和运行介绍可参阅https://mafft.cbrc.jp/alignment/software/。
- 使用hmmbuild对输入的多重比对序列,创建氨基酸水平的隐马尔可夫模型 (HMM) 配置文件,所有结果保存于文件夹hmms中。
使用的代码:hmmbuild $gene.hmm $gene.mafft.fa
注意:HMMER (Eddy, 2011) 软件中hmmbuild的详细参数和运行介绍可参阅http://eddylab.org/software/hmmer/Userguide.pdf。
- 根据HMM配置文件,使用hmmsearch对蛋白质进行结构域搜索BUSCO序列库,每个基因统计结果为$gene.hmmsearch.stats,并保存在文件夹statistics中。
使用的代码:hmmsearch $gene.hmm $gene.mafft.fa > output/$gene.hmm search.out
cat output/$gene.hmmsearch.out | sed -n '15,25p' > statistics/$gene.hmms earch.stats
注意:HMMER软件中hmmsearch的详细参数和运行介绍可参阅http://eddylab.org/software/hmmer/Userguide.pdf。
- 提取每个直同源基因的score,并将BUSCO所需的预期scores_cutoff的临界值定义为HMM搜索中的最小score的90%,最终结果保存至文档scores_cutoffs。
使用的代码:for gene in $(cat candidates.list); do cat statistics/$gene.hmmsearch.stats | awk '{print $2}' | egrep '^[0-9]' > bit_score/$gene.bit_score; done
for gene in $(cat candidates.list); do num1=$(cat bit_score/"$gene".bit_score | sort -k1n | head -n 1); num2=$(echo "scale=2;$num1*0.9"|bc); echo $num2 > lowest_bit_score/$gene.lowest_bit_score; done
for gene in $(cat candidates.list); do sed -i "s/^/"$gene"\t/g" lowest_bit_score/$gene.lowest_bit_score; done
cat lowest_bit_score/* > scores_cutoffs
- 提取每个直同源基因中各个物种的蛋白序列length,将结果统计于lengths,计算标准偏差和平均length分别为lengths后两列,追加最后一列为0,设置BUSCO所需的预期lengths-cutoff。
使用的代码:cat /path/candidates_seqs/$gene.fa | seqkit seq -u -w 0 > 0-raw/$gene.fa
grep -v '^>' 0-raw/$gene.fa > 1-seq/$gene.fa
cat 1-seq/$gene.fa | awk '{print NR "\t" length($0);}' | awk '{print $2}' | sed ':a;N;$!ba;s/\n/ /g' | sed "s/^/"$gene" /g" > 2-length/$gene.length
cat 2-length/* > lengths
cat lengths | awk '{print $1, $16, $14, $15}' | sed "s/ / /g" > lengths_cutoff
注意:可以根据每个基因的标准偏差调整为2倍,降低对提取的BUSCO的长度限制,以增加提取的单拷贝直同源基因数量。
- 使用hmmemit从HMM模型配置文件中得到模式 (祖先) 序列,生成的每个基因的模式序列为$gene.ancestral,合并所有基因的模式序列于文档ancestral中;并允许每个BUSCO产生10条序列并保存为文档$gene.ancestral_variants,合并所有基因的10条序列在文档ancestral_variants中。
使用的代码和参数:hmmemit $gene.hmm > $gene.ancestral
cat * | sed "s/-sample1//g" > ../ancestral
hmmemit -N 10 $gene.hmm > $gene.ancestral_variants
cat *variants | sed "s/-sample/_/g" > ../ancestral_variants
注意:HMMER软件中hmmemit的详细参数和运行介绍可参阅http://eddylab.org/software/hmmer/Userguide.pdf。
- BUSCO评估可以自动调用AUGUSTUS进行基因预测,但需要一个区块配置文件来对每个BUSCO的多重比对序列保守区域进行建模。使用AUGUSTUS中msa2prfl.pl可以将每个直同源基因的多重比对序列转换成所需的配置文件,所有结果保存于文件夹prfl。
使用的代码:msa2prfl.pl $gene.mafft.fa > $gene.prfl
注意:AUGUSTUS软件中msa2prfl.pl的详细参数和运行介绍可参阅https://github.com/nextgenusfs/augustus/blob/master/README.TXT。
- 在新建的USCO数据集collembola_odb1上测试14个弹尾纲物种
使用的代码和参数:run_BUSCO.py -i genome.$SPECIES.fa -c 16 -m geno -o $SPECIES -l /path/collembola_odb1 -sp sinella
注意:BUSCO软件的详细参数和运行介绍可参阅https://busco.ezlab.org/buscouserguide.html。
- (选做) 使用InterProScan (Finn et al., 2017) 针对Pfam、Gene3D、Superfamily和CDD数据库注释所有USCOs的蛋白质结构域,以及基因本体和通路,结果保存至文件夹info。
使用的代码和参数:interproscan.sh -dp -b busco.iprscan -f TSV,GFF3 -goterms -iprlookup -pa -t p -cpu 15 -i /path/collembola_odb1/ancestral -appl Pfam,Gene3D,Superfamily,CDD
注意:InterProScan软件的详细参数和运行介绍可参阅https://interproscan-docs.readthedocs.io/en/latest/。
- 最终的USCO数据集collembola_odb1包含文件及内容见表1。
表1. collembola_odb1包含的文件
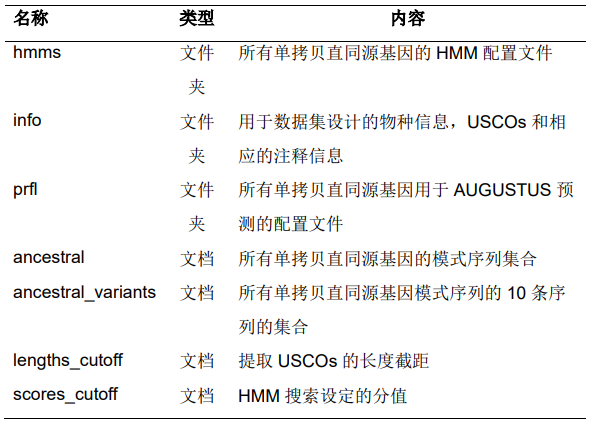
五、UCE设计
我们推荐使用PLWS的Script3 (https://github.com/xtmtd/PLWS/blob/master/script3_UCE_probe_design.sh) 来设计UCE探针,该脚本集成了多种生物信息工具。首先,选取目标类群的基因组数据用于后续标记设计,其中高质量组装的基因组作为参考 (基础) 基因组,主要步骤如下:
- 对于所有的基因组,使用faToTwoBit转换基因组序列fasta格式为.2bit格式,已备后续分析使用。
使用的代码:faToTwoBit $SPECIES/$SPECIES.fasta $SPECIES/${SPECIES%.*}.2bit
注意:faToTwoBit的详细参数和运行介绍可参阅http://hgdownload.soe.ucsc.edu/ admin/exe/。
- 使用ART (Huang et al., 2012) 从基因组中产生每个物种 (不包括参考物种) 长100 bp的成对模拟数据,模拟数据深度$SIMULATE_COV一般设置为2x,可根据类群分歧度适当提高 (一般不超5x),并使用BBTools中reformat.sh进行压缩。
使用的代码和参数:art_illumina --paired --in genomes/$SPECIES/$SPECIES.fasta --out $SPECIES-pe100-reads --len 100 --fcov $SIMULATE_COV --mflen 200 --sdev 50 -ir 0.0 -ir2 0.0 -dr 0.0 -dr2 0.0 -qs 100 -qs2 100 -na
reformat.sh in1=$SPECIES-pe100-reads1.fq in2=$SPECIES-pe100-reads2.fq out=$SPECIES-pe100-reads.fq.gz
注意:ART和BBTools软件的详细参数和运行介绍可分别参阅https://www.niehs.nih.gov/research/resources/software/biostatistics/art/index.cfm和https://sourceforge.net/projects/bbmap/。
- 使用Stampy (Lunter and Goodson, 2011) 将模拟的原始序列比对至参考基因组,生成参考基因组$base的.stidx文件和.sthash文件,碱基替换速率$SUBSTITUTION_RATE通常设置为0.05,可根据类群分歧度适当提高。
使用的代码和参数:python2 stampy.py --species="$base" --assembly="$base" -G $base $base.fasta
python2 stampy.py -g $base -H $base --logfile log
python2 stampy.py --maxbasequal 93 -g $base -h $base --substitutionrate="$SUBSTITUTION_RATE" -t $THREADS --insertsize=200 -M $SPECIES-pe100-reads.fq.gz | samtools view -Sb -@ $THREADS - > $SPECIES-to-$base.bam
注意:Stampy软件的详细参数和运行介绍可参阅http://www.well.ox.ac.uk/project-stampy。
- 使用Samtools (Li et al., 2009) 删除未比对上的reads。
使用的代码和参数:samtools view -h -F 4 -b $SPECIES/$SPECIES-to-$base.bam -@ $THREADS > $SPECIES/$SPECIES-to-$base-MAPPING.bam
注意:Samtools软件的详细参数和运行介绍可参阅http://www.htslib.org/。
- 使用Bedtools (Quinlan and Hall,2010) 将.bam转换为排序的.bed的格式,合并重叠或接近重叠的间隔。
使用的代码和参数:for i in /$SPECIES/$SPECIES-to-$base-MAPPING.bam; do echo $i; bedtools bamtobed -i $i -bed12 > `basename $i`.bed; done
for i in *.bed; do echo $i; bedtools sort -i $i > ${i%.*}.sort.bed; done
for i in *.bam.sort.bed; do echo $i; bedtools merge -i $i > ${i%.*}.merge.bed; done
注意:BEDTools软件的详细参数和运行介绍可参阅https://github.com/arq5x/bedtools2。
- 使用PHYLUCE (Faircloth, 2016) 组件phyluce_probe_strip_masked_loci_from_ set删除所有物种包括参考物种基因组之间的重复间隔。
使用的代码和参数:for i in *.sort.merge.bed; do phyluce_probe_strip_masked_loci_from_set --bed $i --twobit $base.2bit --output ${i%.*}.strip.bed --filter-mask 0.25 --min-length 80; done
注意:PHYLUCE软件的详细参数和运行介绍可参阅http://phyluce.readthedocs.io/en/latest/index.html。 - 创建bed文件的配置文件bed-files.conf,确定共同的保守位点。
使用的代码:phyluce_probe_get_multi_merge_table --conf bed-files.conf --base-taxon $base --output $GROUP-to-$base.sqlite
phyluce_probe_query_multi_merge_table --db $GROUP-to-$base.sqlite --base-taxon $base - 使用PHYLUCE组件phyluce_probe_get_genome_sequences_from_bed从基因组中提取FASTA序列用于候选探针设计。
使用的代码和参数:phyluce_probe_get_genome_sequences_from_bed --bed "$base"+"$SPECIFIC_COUNT".bed --twobit $base.2bit --buffer-to 160 --output "$base"+"$SPECIFIC_COUNT".fasta - 使用PHYLUCE组件phyluce_probe_get_tiled_probes设计临时探针集,$SPECIFIC_COUNT为分类单元数。
使用的代码和参数:phyluce_probe_get_tiled_probes --input "$base"+"$SPECIFIC_COUNT".fasta --probe-prefix "uce-" --design $GROUP-v1 --designer zf --tiling-density 3 --two-probes --overlap middle --masking 0.25 --remove-gc --output "$base"+"$SPECIFIC_COUNT".temp.probes - 使用PHYLUCE组件移除临时探针集中的重复探针。
使用的代码和参数:phyluce_probe_easy_lastz --target "$base"+"$SPECIFIC_COUNT".temp.probes --query "$base"+"$SPECIFIC_COUNT".temp.probes --identity 50 --coverage 50 --output "$base"+"$SPECIFIC_COUNT".temp.probes-TO-SELF-PROBES.lastz
phyluce_probe_remove_duplicate_hits_from_probes_using_lastz --fasta "$base"+"$SPECIFIC_COUNT".temp.probes --lastz "$base"+"$SPECIFIC_COUNT".temp.probes-TO-SELF-PROBES.lastz --probe-prefix=uce- - 将无重复探针的临时探针集与所有基因组进行比对。
使用的代码和参数:phyluce_probe_run_multiple_lastzs_sqlite --probefile "$base"+"$SPECIFIC_COUNT".temp-DUPE-SCREENED.probes --scaffoldlist $(catspecies.list) --genome-base-path genomes/ --identity 50 --cores $THREADS --db "$base"+"$SPECIFIC_COUNT".sqlite --output $GROUP-genome-lastz - 准备基因组数据的配置文件$GROUP-genome.conf,从所有基因组中提取保守位点的序列。
使用的代码和参数:phyluce_probe_slice_sequence_from_genomes --conf $GROUP-genome.conf --lastz $GROUP-genome-lastz --probes 180 --name-pattern ""$base"+"$SPECIFIC_COUNT".temp-DUPE-SCREENED.probes_v_{}.lastz.clean" --output $GROUP-genome-fasta - 使用phyluce_probe_get_multi_fasta_table检测所有基因组中保守位点的一致性,并经phyluce_probe_query_multi_fasta_table统计所有分类单元匹配的分布情况。
使用的代码:phyluce_probe_get_multi_fasta_table --fastas $GROUP-genome-fasta --output multifastas.sqlite --base-taxon $base
phyluce_probe_query_multi_fasta_table --db multifastas.sqlite --base-taxon $base - 输入$FINAL_SPECIFIC_COUNT即分类单元的数量,以确定最终的探针集中将保留多少个基因,比如分类单元数量为10即选择至少10个物种共享的保守基因来设计最终的探针。
使用的代码:phyluce_probe_query_multi_fasta_table --db multifastas.sqlite --base-taxon $base --output "$base"+"$SPECIFIC_COUNT"-back-to-"$FINAL_SPECIFIC_COUNT".conf --specific-counts "$FINAL_SPECIFIC_COUNT" - 设计最终的探针集。
使用的代码和参数:phyluce_probe_get_tiled_probe_from_multiple_inputs --fastas $GROUP-genome-fasta --multi-fasta-output "$base"+"$SPECIFIC_COUNT"-back-to-"$FINAL_SPECIFIC_COUNT".conf --probe-prefix "uce-" --designer zf --design $GROUP-v1 --tiling-density 3 --overlap middle --masking 0.25 --remove-gc --two-probes --output $GROUP-v1-master-probe-list.fasta
- 去除探针集中重复的探针。
使用的代码和参数:phyluce_probe_easy_lastz --target $GROUP-v1-master-probe-list.fasta --query $GROUP-v1-master-probe-list.fasta --identity 50 --coverage 50 --output $GROUP-v1-master-probe-list-TO-SELF-PROBES.lastz
phyluce_probe_remove_duplicate_hits_from_probes_using_lastz --fasta $GROUP-v1-master-probe-list.fasta --lastz $GROUP-v1-master-probe-list-TO-SELF-PROBES.lastz --probe-prefix=uce-
以上所有的UCE探针设计流程详见https://github.com/xtmtd/PLWS/blob/master/script3_UCE_probe_design.sh,可实现一键操作:sh script3_UCE_probe_design.sh
只需准备好基因组数据,并根据提示输入相关内容。
数据分析
根据设计的BUSCO和UCE数据集,我们还提供了相应的标记提取流程,可以通过PLWS中script2_BUSCO_extraction.sh (https://github.com/xtmtd/PLWS/blob/master/script2_BUSCO_extraction.sh) 和script4_UCE_extraction.sh (https://github.com/xtmtd/PLWS/blob/master/script2_BUSCO_extraction.sh) 分别提取USCO和UCE标记。Sun et al. (2020) 根据提取的标记,使用IQ-TREE和ASTRAL进行的系统发育重建结果进一步表明该标记设计方案的有效性。
致谢
该方案中USCO参考标记集和UCE探针设计摘自本课题组已发表的文章Sun et al., 2020. 感谢国家自然科学基金项目 (31970434、31772491) 的资助。
竞争性利益声明
作者声明没有利益冲突。
参考文献
- Zhang, F., Ding, Y., Zhu, C. D., Zhou, X., Orr, M. C., Scheu, S. and Luan, Y. X. (2019). Phylogenomics from low-coverage whole-genome sequencing. Methods Ecol Evol 00: 1-11.
- Sun, X., Ding, Y., Orr, M. C. and Zhang, F. (2020). Streamlining universal single‐copy orthologue and ultraconserved element design: A case study in Collembola. Mol Ecol Resour 20(3): 706-717.
- Bushnell, B. (2014). BBtools. https://sourceforge.net/projects/bbmap/
- Chikhi, R. and Rizk, G. (2013). Space-efficient and exact de Bruijn graph representation based on a Bloom filter. Algorithms Mol Biol 8: 22.
- Bankevich, A., Nurk, S., Antipov, D., Gurevich, A. A., Dvorkin, M., Kulikov, A. S., Lesin, V. M., Nikolenko, S. I., Pham, S., Prjibelski, A. D., Pyshkin, A. V., Sirotkin, A. V., Vyahhi, N., Tesler, G., Alekseyev, M. A. and Pevzner, P. A. (2012). SPAdes: a new genome assembly algorithm and its applications to single-cell sequencing. J Comput Biol 19(5): 455-477.
- Pryszcz, L. P. and Gabaldón, T. (2016). Redundans: an assembly pipeline for highly heterozygous genomes. Nucleic Acids Res 44: e113.
- Li, H. (2018). Minimap2: pairwise alignment for nucleotide sequences. Bioinformatics 34: 3094-3100.
- Sahlin, K., Vezzi, F., Nystedt, B., Lundeberg, J. and Arvestad, L. (2014). BESST–efficient scaffolding of large fragmented assemblies. BMC Bioinformatics 15: 281.
- Luo, R., Liu, B., Xie, Y., Li, Z., Huang, W., Yuan, J., He, G., Chen, Y., Pan, Q., Chen, Y., Liu, Y., Tang, J., Wu, G., Zhang, H., Shi, Y., Liu, Y., Yu, C., Wang, B., Lu, Y., Han, C., Cheung, D. W., Yiu, S. M., Peng, S., Zhu, X., Liu, G., Liao, X., Li, Y., Yang, H., Wang, J. and Lam, T. W. (2012). SOAPdenovo2: an empirically improved memory-efficient short-read de novo assembler. Gigascience 1: 18.
- Simão, F. A., Waterhouse, R. M., Ioannidis, P., Kriventseva, E. V. and Zdobnov, E. M. (2015). BUSCO: assessing genome assembly and annotation completeness with single-copy orthologs. Bioinformatics 31: 3210-3212.
- Morgulis, A., Gertz, E. M., Schaffer, A. A. and Agarwala, R. (2006). WindowMasker: Window based masker for sequence genomes. Bioinformatics 22 (2): 134-141.
- Bruna, T., Hoff, K. J., Lomsadze, A., Stanke, M. and Borodovsky, M. (2015). BRAKER2: automatic eukaryotic genome annotation with GeneMark-EP+ and AUGUSTUS supported by a protein database. NAR Genomics Bioinf 3(1): lqaa108.
- Buchfink, B., Xie. C. and Huson. D. H. (2015). Fast and sensitive protein alignment using DIAMOND. Nat Methods 12(1): 59-60.
- Fu, L., Niu, B., Zhu, Z., Wu, S. and Li, W. (2012). CD-HIT: accelerated for clustering the next generation sequencing data. Bioinformatics 28(23): 3150-3152.
- Emms, D. M. and Kelly, S. (2019). OrthoFinder: phylogenetic orthology inference for comparative genomics. Genome Biol 20: 238.
- Katoh, K. and Standley, D. M. (2013). MAFFT multiple sequence alignment software version 7: Improvements in performance and usability. Mol Biol Evol 30: 772-780.
- Eddy, S. R. (2011). Accelerated profile HMM searches. PLoS Comput Biol 7(10): e1002195.
- Finn, R. D., Attwood, T. K., Babbitt, P. C., Bateman, A., Bork, P., Bridge, A. J., Chang, H. Y., Dosztányi, Z., El-Gebali, S., Fraser, M., Gough, J., Haft, D., Holliday, G. L., Huang, H., Huang, X., Letunic, I., Lopez, R., Lu, S., Marchler-Bauer, A., Mi, H., Mistry, J., Natale, D. A., Necci, M., Nuka, G., Orengo, C. A., Park, Y., Pesseat, S., Piovesan, D., Potter, S. C., Rawlings, N. D., Redaschi, N., Richardson, L., Rivoire, C., Sangrador-Vegas, A., Sigrist, C., Sillitoe, I., Smithers, B., Squizzato, S., Sutton, G., Thanki, N., Thomas, P. D., Tosatto, S. C. E., Wu, C. H., Xenarios, I., Yeh, L. S., Young, S. Y. and Mitchell, A. L. (2017). InterPro in 2017-beyond protein family and domain annotations. Nucleic Acids Res 45: D190-D199.
- Huang, W., Li, L., Myers, J. R. and Marth, G. T. (2012). ART: a next-generation sequencing read simulator. Bioinformatics 28: 593-594.
- Lunter, G. and Goodson, M. (2011). Stampy: a statistical algorithm for sensitive and fast mapping of Illumina sequence reads. Genome Res 21: 936-939.
- Li, H., Handsaker, B., Wysoker, A., Fennell, T., Ruan, J., Homer, N., Marth, G., Abecasis, G. and Durbin, R. (2009). 1000 genome project data processing subgroup. The sequence alignment/map (SAM) format and SAMtools. Bioinformatics 25(16): 2078-2079.
- Quinlan, A. R. and Hall, I. M. (2010). BEDTools: a flexible suite of utilities for comparing genomic features. Bioinformatics 26: 841-842.
- Faircloth, B. C. (2016). PHYLUCE is a software package for the analysis of conserved genomic loci. Bioinformatics 32: 786-788.
Copyright: © 2021 The Authors; exclusive licensee Bio-protocol LLC.
引用格式:丁银环, 杜诗雨, 张峰. (2021). 利用基因组数据设计通用单拷贝核基因与超保守元件分子标记方法.
Bio-101: e1010632. DOI:
10.21769/BioProtoc.1010632.
How to cite: Ding, Y. H., Du, S. Y. and Zhang, F. (2021). Streamlining Universal Single-copy Orthologue and Ultraconserved Element Design Method from Whole Genome Sequencing.
Bio-101: e1010632. DOI:
10.21769/BioProtoc.1010632.